Layers of artificial neural networks and their role in clinical decision support
Article by Master, Doctor Mai Vien Phuong - Gastrointestinal endoscopist - Department of Medical Examination & Internal Medicine - Vinmec Central Park International General Hospital.
Accumulating evidence shows that the application of artificial neural networks can help overcome treatment limitations caused by individual differences and batch data. Therefore, chemotherapy sensitivity assessment with artificial neural network models is a hot topic.
1. Overview of artificial neural networks (neurons)
The concept of artificial neural network was first proposed in 1943. The development of artificial neural network models has approached in a complex manner since then. During the 1980s, the rapid advancement of artificial neural network algorithms spurred a modern revolution. The design of the artificial neural network is based on the neural network of the human brain, which is mainly composed of many neurons. Streams of data (i.e. signals) are transmitted and programmed through the neural nodes. Nerve cells in different layers have their own task to solve problems, possibly similar to factory production lines. Deconstructing tasks for data analysis provides the ability to find the optimal solution in the shortest time.
As a type of parallel distributed system driven by large volumes of data, artificial neural networks are not subject to the requirements of known logical or mathematical associations.
2. Structure of an artificial neural network
The simplest artificial neural network is a single-layer network (i.e. a perceptron neural network) and has an input layer, an output layer, and a hidden layer. Single-layer networks can only be used to describe a dichotomy based on a single factor, thus rendering most of the problems out of its reach. Artificial neural network depth amplification can enhance the ability to fit geometrically complex features. Researchers began to create artificial neural network models with many layers. Even in 2016, He et al. reported an artificial neural network model with more than 1000 layers. However, excessive neural network depth can lead to system instability and loss of superficial learning.
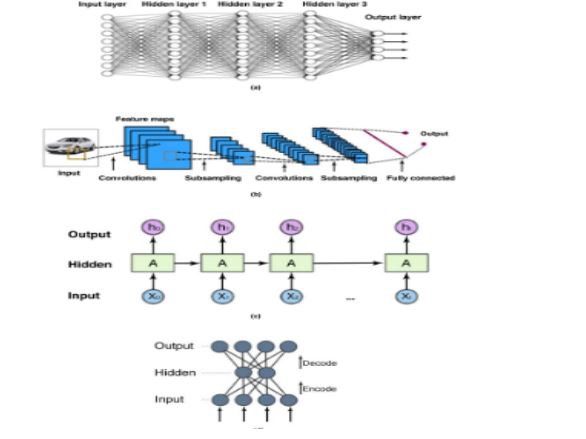
Cấu trúc các lớp một mạng nơron nhân tạo.
3. Classification of Artificial Neural Networks Artificial neural networks can be divided into two main categories based on the direction of signal flow between hidden layers.
There are many differences between the two types of artificial neural networks. In a feed-forward neural network (FNN), the data flow is unidirectional from top to bottom with no feedback; no connection exists between neurons in any given layer. In terms of systems learning, FNN is convenient for reprogramming and can handle non-linear questions. The other is the feedback neural network. A neuron in a feedback neural network can transmit its output data to other neurons in the same or upper layers. The algorithms will be simultaneously tuned by signals from other neurons based on the pre-knowledge data set. The repeated calibration contributes to the excellent strength and accuracy of the artificial neural network. Feedback neural networks are commonly used for image analysis, diagnosis, and prediction of outcomes.
There are many differences between the two types of artificial neural networks. In a feed-forward neural network (FNN), the data flow is unidirectional from top to bottom with no feedback; no connection exists between neurons in any given layer. In terms of systems learning, FNN is convenient for reprogramming and can handle non-linear questions. The other is the feedback neural network. A neuron in a feedback neural network can transmit its output data to other neurons in the same or upper layers. The algorithms will be simultaneously tuned by signals from other neurons based on the pre-knowledge data set. The repeated calibration contributes to the excellent strength and accuracy of the artificial neural network. Feedback neural networks are commonly used for image analysis, diagnosis, and prediction of outcomes.
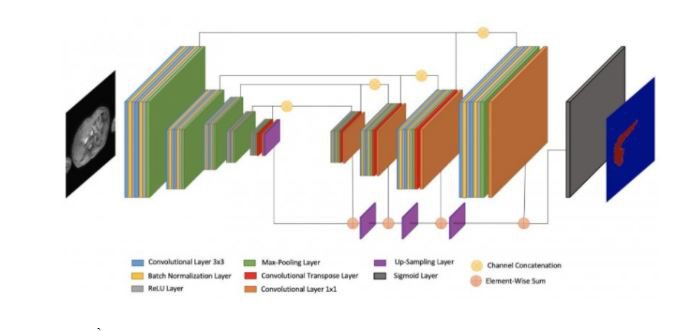
Các luồng tín hiệu được xử lí trong công nghệ AI
Besides, the "one-to-many" relationship between neurons ensures the learning efficiency of the artificial neural network.
A layer of neurons can simultaneously transmit signals to more than one neuron. It is important that the signal flow is distributed unevenly to each neuron in the next layer. The flow inequality makes the artificial neural network able to adjust to the external environment. Before calculating the activation functions, the data will be adjusted for weighting factors, each of which has a corresponding relationship with a particular neuron.
The weight factor acts as the data flow controller. Larger weight factors have a greater influence on the output. Conversely, setting the weighting factor to zero can replace the functions of the corresponding neuron.
4. Artificial Neural Networks and Role in Clinical Decision Support Clinical decision making plays a concluding role in the process. Many doctors and scientists have been trying to promote standardized, precise and personalized treatment. As the main adjuvant regimen for gastrointestinal cancer, chemotherapy should consider many factors, such as patient tolerance, pathologic sensitivity, specific dosage, and regimen.
Accumulating evidence shows that the application of artificial neural networks can help overcome treatment limitations caused by individual differences and batch data. Evaluation of chemotherapy sensitivity with artificial neural network models is a hot topic. To establish reliable prediction systems for locally advanced and metastatic colorectal cancer, several artificial neural network models have been used to integrate clinical indicators. Their accuracy was significantly better than that of clinicians.
Furthermore, deep learning on radiographic imaging shows its potential value in the assessment of chemotherapy. For example, a CNN system was trained using 202 cases of colorectal cancer with liver metastases and was found to have good accuracy in predicting response to FOLFOX in combination with the bevacizumab regimen. based on CT information. The feasibility of radiation therapy, anti-integral therapy, traditional Chinese medicine and immunotherapy can also be improved with the help of artificial neural networks.
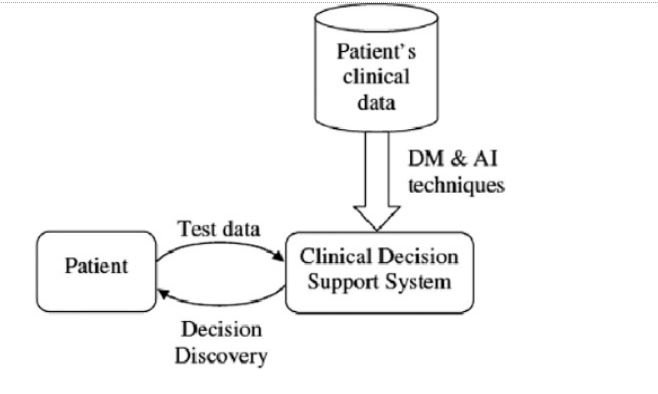
Tổng hợp các dữ liệu giúp trí tuệ nhân tạo đưa ra các quyết định điều trị
5. Artificial neural networks for stage recognition and surgical quality control Quality control of surgical guidance and supervision is highly dependent on the skill of the clinician. It is difficult to train normally trained physicians and ensure surgical procedures are not interfered with by factors of uncertainty. Kitaguchi and colleagues created a new deep learning method based on CNN powered by intraoperative videos. It can automatically recognize the surgical stage and act with high accuracy of 81% and 83.2% respectively. These achievements will start a new field of application of artificial neural networks. Clinicians, especially young surgeons, will reap many benefits from these technological innovations. Unfortunately, some studies have been reported due to ethical limitations and complicated surgical situations. Greater care and investment is needed to develop effective guidance systems that precisely match the surgeon's skills and practices.
Để đặt lịch khám tại viện, Quý khách vui lòng bấm số HOTLINE hoặc đặt lịch trực tiếp TẠI ĐÂY. Tải và đặt lịch khám tự động trên ứng dụng MyVinmec để quản lý, theo dõi lịch và đặt hẹn mọi lúc mọi nơi ngay trên ứng dụng.
References:
Cao B, Zhang KC, Wei B, Chen L. Status quo and future prospects of artificial neural network from the perspective of gastroenterologists. World J Gastroenterol 2021; 27(21): 2681-2709 [DOI: 10.3748/wjg.v27.i21.2681]
Mungle T , Tewary S, Das DK, Arun I, Basak B, Agarwal S, Ahmed R, Chatterjee S, Chakraborty C. MRF -ANN: a machine learning method for automated ER scoring on breast cancer immunohistochemistry images. J Microsc . Year 2017; 267 : 117-129. [ PubMed ] [ DOI ] [ Quoted in this article: 1 ]
Bài viết này được viết cho người đọc tại Sài Gòn, Hà Nội, Hồ Chí Minh, Phú Quốc, Nha Trang, Hạ Long, Hải Phòng, Đà Nẵng.