Risk stratification and prognostic prediction of GISTs by data visualization techniques
Post by Master, Doctor Mai Vien Phuong - Department of Examination & Internal Medicine - Vinmec Central Park International General Hospital
Gastrointestinal stromal tumors (GISTs) are uncommon mesenchymal tumors of the gastrointestinal tract, originating from the interstitial cells of Cajal. GISTs can arise anywhere along the gastrointestinal tract, most commonly in the stomach (50%-60% of cases) or the small intestine (30%-40%), while they are rarely observed in the gastrointestinal tract. esophagus and colon.
All GISTs are potentially malignant with varying degrees of biological aggression. Contrast computed tomography (CT) is the imaging modality of choice for preoperative diagnosis, staging, as well as postoperative follow-up and assessment of treatment response in patients with GISTs.
Datagrammetry is based on mathematical quantification of image heterogeneity, through analysis of the distribution and relationship of pixel intensities in a region of interest (ROI). Analysis of data-forming images requires a multi-step process, starting with image acquisition, and including lesion segmentation, feature extraction, feature selection and reduction, building predictive models, and ultimately validate and interpret clinical results.
Datagrammetry is based on mathematical quantification of image heterogeneity, through analysis of the distribution and relationship of pixel intensities in a region of interest (ROI). Analysis of data-forming images requires a multi-step process, starting with image acquisition, and including lesion segmentation, feature extraction, feature selection and reduction, building predictive models, and ultimately validate and interpret clinical results.
1. Risk stratification and prognostic prediction of GISTs by data visualization techniques
Accurate assessment of malignancy risk and outcome in GISTs is primarily based on tumor size, location (gastric versus extragastric tumor), and mitotic count obtained from resected specimens. These factors were combined in the 2008 National Institutes of Health criteria, which classified GISTs into four risk categories: very low, low, moderate, and high tumor risk. However, in clinical practice, risk stratification can be limited by assessing mitotic counts in patients treated with new adjuvant therapy, or by evaluating biopsy specimens. cannot represent the entire tumor. Therefore, several studies have attempted to predict risk stratification based on preoperative CT imaging. CT features such as size, growth pattern, or enlarged feeding vessels are associated with high-risk tumors. However, risk stratification using qualitative image assessments is influenced by reader experience, heterogeneous definitions of imaging features, and subjective assessments with underreproducibility. optimization of qualitative characteristics
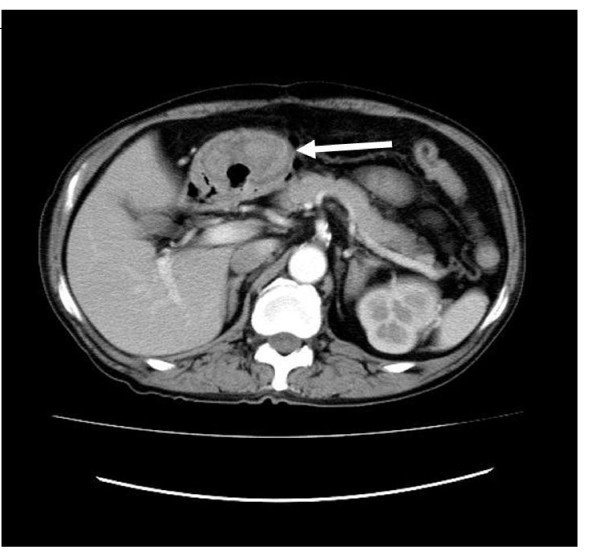
Dự đoán phân tầng u mô đệm đường tiêu hóa qua ảnh CT
2. Datagram imaging models have demonstrated that improved preoperative prediction of high-risk GIST compared with conventional imaging assessment
The added value of angiography and texture analysis on contrast-enhanced CT was first studied by Yan et al in a retrospective cohort of 213 small bowel GISTs. In that study, the texture analysis model achieved similar diagnostic accuracy with respect to the clinical and subjective imaging features for predicting the preoperative risk of GISTs. When combining the texture and clinical analysis features, the diagnostic performance (AUROC of 0.943) is significantly improved compared with the model that only combines clinical and imaging features. In a more recent study, Choi et al investigated the diagnostic performance of histogram-based texture parameters and qualitative analysis of CT imaging features to distinguish low-risk from high-risk GIST. High. Their results confirm that the data visualization features show a higher diagnostic performance (AUROC of 0.782-0.779) than the conventional qualitative assessment (AUROC of 0.59-0.70) by Two physicians Datagrammetry in the differential diagnosis of low-risk from high-risk GISTs.
3. The potential of data visualization
The potential of data visualization for risk stratification in GISTs has been further evaluated by other evidence with promising results and excellent diagnostic efficacy. Liu et al applied CT-based structural analysis to identify very low and low-risk GISTs in a cohort of 78 patients, reporting a fair diagnosis (AUROC of 0.637-0.811) for the The most distinguishing features are obtained on anterior contrast, arterial and venous phases of CT images. Feng et al extracted histogram-based parameters from arterial and venous phase CT images of 90 small bowel GISTs. Among them, entropy showed the highest diagnostic accuracy on the arterial and venous phases (AUROC of 0.823 and 0.830, respectively) for identifying high-risk GISTs. Zhang et al analyzed 140 GISTs using arterial stage CT imaging, reporting excellent diagnostic performance for preoperative prediction of advanced GIST (i.e., high and moderate risk) and stratification. four-class risk (AUROC 0.935 and 0.809, respectively). Differentiate low-risk GIST from high-risk GIST
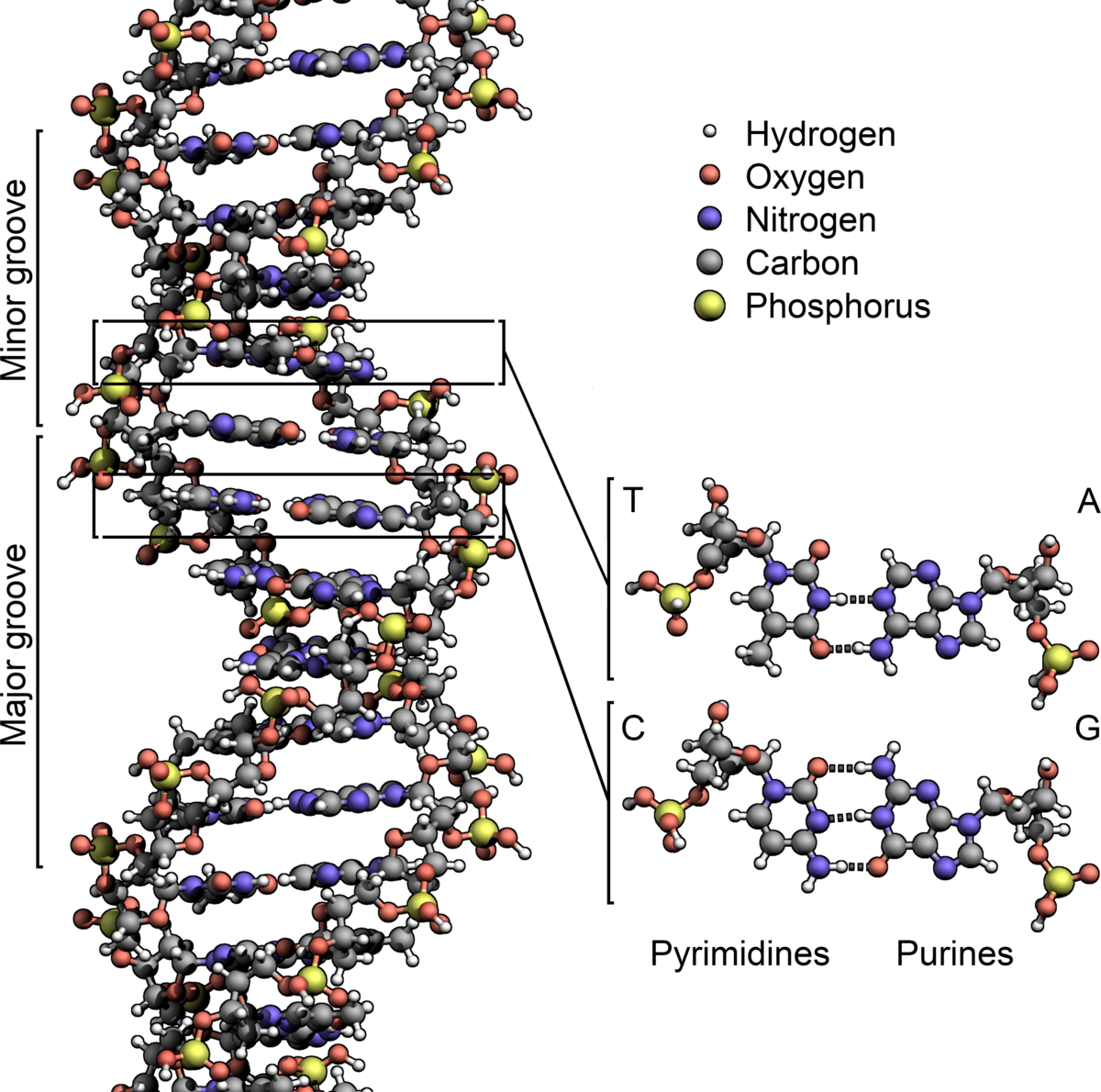
Tạo hình dữ liệu hóa giúp phân biệt u mô đệm đường tiêu hóa cao thấp
In a large population of 440 pathologically proven GISTs, Ren et al reported an excellent performance of data visualization models to distinguish low-risk GIST from high-risk GIST. high muscle (AUROC was 0.935 and 0.933 in the training and validation groups, respectively). In that study, predictive imaging (combining lesion size, cystic degeneration, and structure-based median) showed a sensitivity of 90.6% and specificity of 75.7% for the diagnosis of GISTs. high risk. Similarly, Wang et al analyzed the contrast-enhanced CT images of 333 GISTs and reported the excellent discriminating ability of the Datagram Imaging models between low-risk and high-risk GIST in both training and validation groups (AUROC 0.882 and 0.920, respectively). In addition, data visualization models allow to differentiate GISTs with low and high mitotic counts with good to excellent performance (AUROC: 0.769-0.820).
Prediction of malignancy potential or postoperative recurrence-free survival In two subsequent studies, Chen et al built a support vector machine and a residual neural network-based model to predict potential malignancy or recurrence-free survival 3 years and 5 years after focal total resection of GIST, respectively. In those studies, the Authors enrolled a cohort of medical patients for model training, which was then validated in internal and external groups, with performance ranging from good to close. as perfect for GIST risk stratification and prediction of recurrence-free survival at 3 years and 5 years, respectively, Structural feature survival analysis of disease progression was also performed. by Ekert et al. on contrast-enhanced CT, whereas only one study performed data visualization on MRI. Fu et al extracted texture features from T2, DWI and ADC weighted map images to determine the prognosis of metastatic GIST, reporting that texture features on DWI and ADC maps have correlated well with overall survival.
Finally, the Ki67 index represents a marker of tumor cell proliferation, which is also associated with poor prognosis in GISTs. In a study of 339 GISTs, the Contrast-enhanced CT angiography marker demonstrated a significant correlation with Ki67 expression, providing an additional value for prognostic assessment.
Prediction of malignancy potential or postoperative recurrence-free survival In two subsequent studies, Chen et al built a support vector machine and a residual neural network-based model to predict potential malignancy or recurrence-free survival 3 years and 5 years after focal total resection of GIST, respectively. In those studies, the Authors enrolled a cohort of medical patients for model training, which was then validated in internal and external groups, with performance ranging from good to close. as perfect for GIST risk stratification and prediction of recurrence-free survival at 3 years and 5 years, respectively, Structural feature survival analysis of disease progression was also performed. by Ekert et al. on contrast-enhanced CT, whereas only one study performed data visualization on MRI. Fu et al extracted texture features from T2, DWI and ADC weighted map images to determine the prognosis of metastatic GIST, reporting that texture features on DWI and ADC maps have correlated well with overall survival.
Finally, the Ki67 index represents a marker of tumor cell proliferation, which is also associated with poor prognosis in GISTs. In a study of 339 GISTs, the Contrast-enhanced CT angiography marker demonstrated a significant correlation with Ki67 expression, providing an additional value for prognostic assessment.
Để đặt lịch khám tại viện, Quý khách vui lòng bấm số HOTLINE hoặc đặt lịch trực tiếp TẠI ĐÂY. Tải và đặt lịch khám tự động trên ứng dụng MyVinmec để quản lý, theo dõi lịch và đặt hẹn mọi lúc mọi nơi ngay trên ứng dụng.
References Cannella R, La Grutta L, Midiri M, Bartolotta TV. New advances in scintigraphy of gastrointestinal stromal tumors. World J Gastroenterol 2020; 26 (32): 4729-4738 [PMID: 32921953 DOI: 10.3748 / wjg.v26.i32.4729 ]
Bài viết này được viết cho người đọc tại Sài Gòn, Hà Nội, Hồ Chí Minh, Phú Quốc, Nha Trang, Hạ Long, Hải Phòng, Đà Nẵng.