Artificial intelligence AI for the diagnosis of polyps and colon cancer
The article is written by MSc, BS. Mai Vien Phuong, Department of Medical Examination & Internal Medicine - Vinmec Central Park International General Hospital
AI AI for computer-aided diagnosis of upper gastrointestinal endoscopy is increasingly gaining attention for automatic and accurate identification of dysplasia in Barrett's esophagus, as well as for cancer detection. early gastric cancer (GCs), thus preventing malignancies of the esophagus and stomach.
1. Overview of artificial intelligence
Artificial intelligence (AI) enables machines to provide disruptive value in a number of industries and applications. Applications of AI techniques, especially machine learning and more recently deep learning, are growing in gastroenterology. Computer-aided diagnosis of upper gastrointestinal endoscopy is increasingly attracting attention for the automatic and accurate identification of dysplasia in Barrett's esophagus, as well as for the early detection of gastric cancers (GCs). , thus preventing malignancies in the esophagus and stomach. In addition, sophisticated neural network technology can accurately assess Helicobacter pylori (H. pylori) infection during standard endoscopy without biopsy, thereby reducing the risk of cancer stomach letter. Artificial intelligence has the potential to be applied during colonoscopy to automatically detect colorectal polyps and distinguish between cancerous and noncancerous polyps, with the potential to improve detection rates. adenomas, the incidence varies widely among endoscopists when performing a colonoscopy examination. In addition, AI allows to establish the feasibility of endoscopic polypectomy of large colon polyp lesions based on surface features and microvascular changes
2. Introduction to machine learning algorithms and deep learning
Artificial intelligence (AI) is based on artificial intelligence elements that perform functions related to the human mind, such as learning and problem solving. In endoscopy, AI has begun to aid in improving colon polyp detection and adenoma detection (ADR) rates, to distinguish between benign and precancerous lesions based on the interpretation of surface patterns. their faces.
Machine learning (ML) and deep learning (DL) can be considered as subfields of artificial intelligence. ML is a form of artificial intelligence that can support decision-making, allowing improvements to applied algorithms without programming, including examining data and implementing descriptive models and models. forecast.
Machine learning (ML) and deep learning (DL) can be considered as subfields of artificial intelligence. ML is a form of artificial intelligence that can support decision-making, allowing improvements to applied algorithms without programming, including examining data and implementing descriptive models and models. forecast.
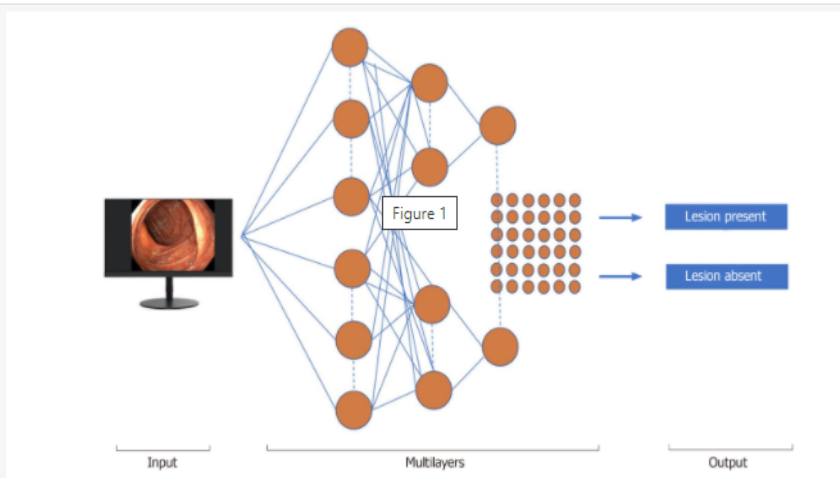
Mô hình ứng dụng trí tuệ nhân tạo trong nội soi tiêu hoá
Machine learning (ML) algorithms are differentiated into supervised and unsupervised methods. An example of supervised machine learning technology, an artificial neural network (ANN), mirrors the brain's schematic function. Each neuron is a computational unit and all neurons are connected to create a network. Machine learning algorithms and complex neural networks (CNNs) were created to train the software to distinguish between normal and abnormal regions in the intestinal lumen. To detect polyps, machine learning uses a fixed number of characteristics, such as the size, shape, and mucosal patterns of polyps.
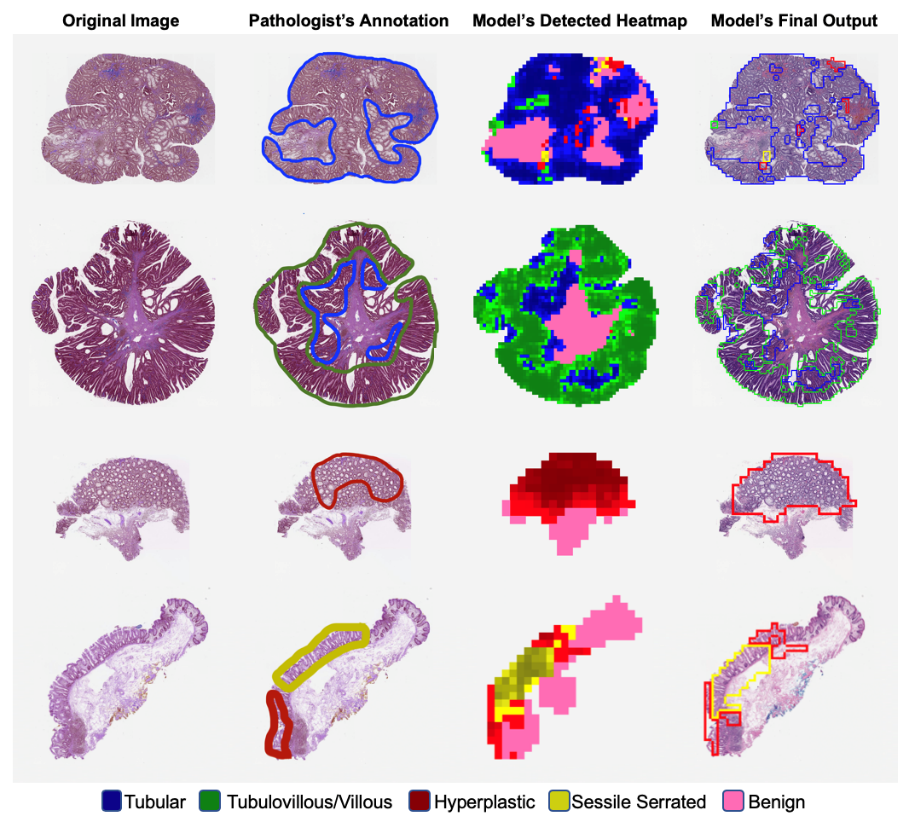
Mô hình máy tính phân tích để đánh giá khả năng ung thư của polyp đại tràng
3. AI AI for diagnosing polyps and colon cancer
Colorectal cancer (CRC) is the third most common malignancy in men and the second in women, and the fourth most common cause of cancer death. The National Polyp Study Registered that 70%-90% CRC can be prevented with routine endoscopic monitoring and polyp removal, but 7%-9% CRC can occur regardless of measures. this.
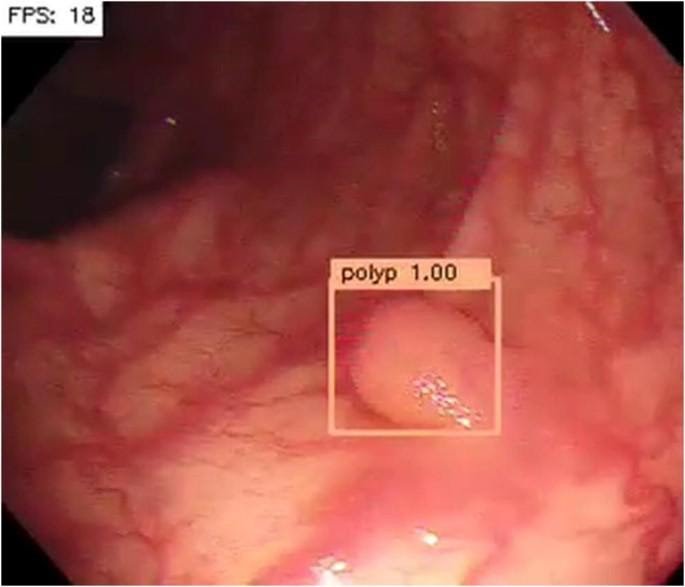
Hình ảnh nội soi có sử dụng trí tuệ nhân tạo: máy tính tự động tìm và định vị polyp, đánh giáp polyp đó bao nhiêu phần trăm ung thư
About 85% of "missed cancers" are caused by missed polyps or incomplete resection of polyps. Adenomas are the most common precancerous lesions throughout the colon. The ADR measures the endoscopist's ability to identify adenomas. ADRs range from 7%–53% among performing endoscopists depending on their training, laparoscopic resection technique, time of withdrawal, quality of bowel preparation, and dependent determinants. into another process.
Several endoscopic improvements have been promoted to increase ADR. A review of 5 studies on the effects of high-resolution colonoscopy on ADR showed conflicting results; One study concluded that the ADR was elevated exclusively for endoscopists with an ADR lower than 20%.
Several endoscopic improvements have been promoted to increase ADR. A review of 5 studies on the effects of high-resolution colonoscopy on ADR showed conflicting results; One study concluded that the ADR was elevated exclusively for endoscopists with an ADR lower than 20%.
4. Application of the complex neural network system in the diagnosis of colon polyps
Computer-aided diagnostic (CAD) system analysis has the potential to aid in the detection of additional adenomas. Urban et al., used a set of 8641 different and representative hand-labelled images from screening bronchoscopes processed on 2000 patients. They tested the models on 20 endoscopic videos with a total duration of 5 hours. Colonoscopy specialists were asked to identify all polyps in 9 unidentified colonoscopy videos, selected from archived video studies, with/without benefit of CNN overlay. Their findings correlated with CNN's results using a CNN-supported expert review as a reference. CNN identified polyps with an AUC of 0.99 and an accuracy of 96.4%. Indeed, in a colonoscopy video analysis involving 28 polyps removed, 4 reviewers identified 8 more polyps (omitted) without CNN assistance and recognized 17 more polyps with support from CNN. CNN detected all polyps removed and recognized by expert review, a false positive rate of 7%. This strategy may improve ADR and reduce cancer interval but it requires further studies to be fully implemented. AI can be used during endoscopic evaluation to automatically recognize colorectal polyps and distinguish between malignant and non-malignant lesions. CAD is based on the time delay from acquiring an image to processing it for the final image on the screen. This model can detect polyps with a sensitivity of 96.5%. A recent RCT estimated the impact of an automated polyp detection system based on a deep learning algorithm during real-time colonoscopy. This study involving 1058 patients demonstrated that the AI system enhanced ADR by almost 10%.
A prospective study of 55 patients used the prototype of a new automated polyp detection software (APDS) for automated image-based polyp detection and with overall polyp detection rates over time. actually 75%. Smaller polyp size and flat polyp morphology are associated with APDS not detecting enough polyps.
A prospective study of 55 patients used the prototype of a new automated polyp detection software (APDS) for automated image-based polyp detection and with overall polyp detection rates over time. actually 75%. Smaller polyp size and flat polyp morphology are associated with APDS not detecting enough polyps.
5. AI's ability to distinguish between adenomas and hyperplastic polyps
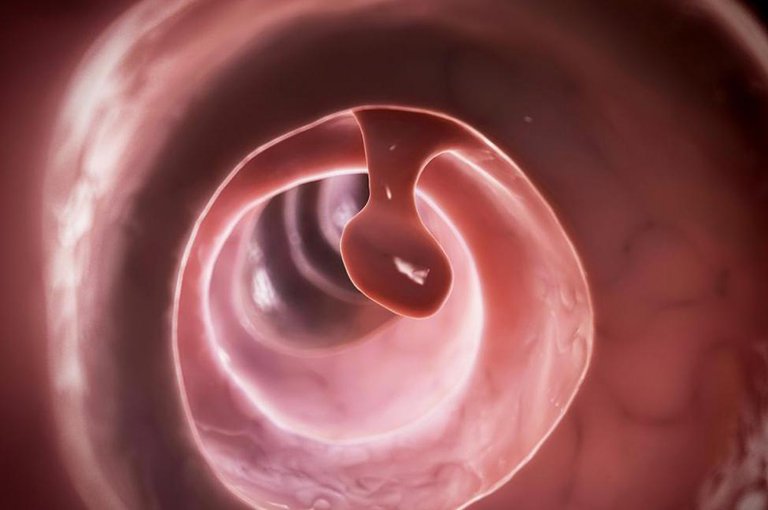
Ngoài máy móc CADe, CADx còn được sử dụng để phân biệt giữa u tuyến và polyp tăng sản
In addition to the CADe machine, CADx is also used to differentiate between adenomas and hyperplastic polyps. Byrne et al proposed the use of computerized image analysis to reduce variability in endoscopic findings and histological predictions. This AI model was trained using endoscopic video and was able to distinguish between small adenomas and hyperplastic polyps with high accuracy. In addition, it predicted histology with 94% accuracy, 98% sensitivity, 83% specificity, and negative and positive predictive values of 97% and 90%, respectively.
Furthermore, an AI-assisted image classifier, based on non-optical magnifying endoscopic NBI, was used to predict the histology of isolated colonic lesions, after evaluating 3509 colon damage. The most common histologic types were ductal adenomas (47.6%), deeply invasive carcinoma (15.9%), superficially invasive carcinoma (7.9%), and raised polyps. obstetrics (14.3%), sessile serrated polyps (7.9%) and tubular adenomas (6.6%). The sensitivity of hyperplastic and serrated polyps was 96.6%, although this rate was lower for ductal adenomas and cancers. When investigating only small colonic polyps, the correlation of the duration of surveillance colonoscopy using AI and histological image classification was 0.97. Furthermore, this classifier also showed high accuracy (88.2%) in predicting deep invasive, endoscopically incurable carcinoma, HNPV, and accuracy for carcinoma. Deeply invasive tissue has also been shown to aid in the selection of treatable lesions.
Furthermore, an AI-assisted image classifier, based on non-optical magnifying endoscopic NBI, was used to predict the histology of isolated colonic lesions, after evaluating 3509 colon damage. The most common histologic types were ductal adenomas (47.6%), deeply invasive carcinoma (15.9%), superficially invasive carcinoma (7.9%), and raised polyps. obstetrics (14.3%), sessile serrated polyps (7.9%) and tubular adenomas (6.6%). The sensitivity of hyperplastic and serrated polyps was 96.6%, although this rate was lower for ductal adenomas and cancers. When investigating only small colonic polyps, the correlation of the duration of surveillance colonoscopy using AI and histological image classification was 0.97. Furthermore, this classifier also showed high accuracy (88.2%) in predicting deep invasive, endoscopically incurable carcinoma, HNPV, and accuracy for carcinoma. Deeply invasive tissue has also been shown to aid in the selection of treatable lesions.
6. Role of AI in lesion assessment before performing mucosal EMR
We also evaluated the use of an AI-assisted image classifier in determining the feasibility of EMR mucosal resection of large colonic lesions based on unmagnified imaging. The independent test kit included 76 colonic lesions that met the indications for laparoscopic submucosal surgery. Overall, the trained AI image classifier showed a sensitivity of 88.2% (95% CI: 84.7-91.1%). cured with a specificity of 77.9% (95% CI: 70.3-84.4%) and an accuracy of 85.5% (95% CI: 82.4-88.3%). This study determines the high accuracy of a trained AI image classifier in predicting the feasibility of mucosal resection for large colonic lesions. While the advancement of AI using CNN is great for the recognition of specific mucosal patterns and image classification, in the next future the prediction performance could be superior to that of a specialized endoscopist. Karma.
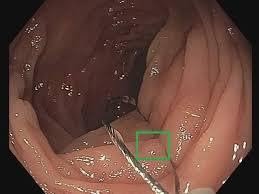
Cắt polyp bằng thủ thuật EMR có sự hỗ trợ của trí tuệ nhân tạo
7. The role of the endoscopic (EC) - CAD system in the diagnosis of colorectal lesions
Hotta et al aimed to confirm the effectiveness of the endoscopic (EC)-CAD system in the diagnosis of malignant or non-malignant colorectal lesions, by comparing the diagnostic capabilities among professional endoscopists. and non-experts, using web-based tests. A confirmatory test was performed using endoscopic images of 100 small (<10 mm) colorectal lesions. The diagnostic accuracy and sensitivity of EB-01 and non-specialists for stained endoscopic images was 98.0% versus 69.0%, indicating significantly higher diagnostic accuracy and sensitivity for with non-specialist endoscopists when diagnosing small colorectal lesions.
A prospective, open-label, one-group study evaluated the performance of real-time EC-CAD in 791 consecutive patients undergoing colonoscopy and 23 endoscopic surgeons to differentiate neoplastic polyps (adenomas). requires non-cancerous polyp removal that requires no treatment, potentially reducing costs. The results showed a negative predictive value of 96.4% of CAD with staining mode in the best case while 93.7% in the worst case. Clever using NBI, 96.5% and 95.2% best case and worst case.
Finally, in a study including 117 patients with stage IIA colorectal cancer after radical surgery, the ANN-based scoring system, based on tumor molecular characteristics, recognized those had a high, moderate, and low probability of survival at the 10-year follow-up period. The 10-year overall survival rates were 16.7%, 62.9%, and 100% (P < 0.001), while the 10-year disease-free survival rates were 16.7%, 61.8%, and 98.8, respectively. %. This study reveals that the scoring system gives individuals at high risk of stage IIA colorectal cancer for a more aggressive treatment.
A prospective, open-label, one-group study evaluated the performance of real-time EC-CAD in 791 consecutive patients undergoing colonoscopy and 23 endoscopic surgeons to differentiate neoplastic polyps (adenomas). requires non-cancerous polyp removal that requires no treatment, potentially reducing costs. The results showed a negative predictive value of 96.4% of CAD with staining mode in the best case while 93.7% in the worst case. Clever using NBI, 96.5% and 95.2% best case and worst case.
Finally, in a study including 117 patients with stage IIA colorectal cancer after radical surgery, the ANN-based scoring system, based on tumor molecular characteristics, recognized those had a high, moderate, and low probability of survival at the 10-year follow-up period. The 10-year overall survival rates were 16.7%, 62.9%, and 100% (P < 0.001), while the 10-year disease-free survival rates were 16.7%, 61.8%, and 98.8, respectively. %. This study reveals that the scoring system gives individuals at high risk of stage IIA colorectal cancer for a more aggressive treatment.
8. Role of AI in differentiating patients with complete response to adjuvant chemotherapy for advanced rectal cancer
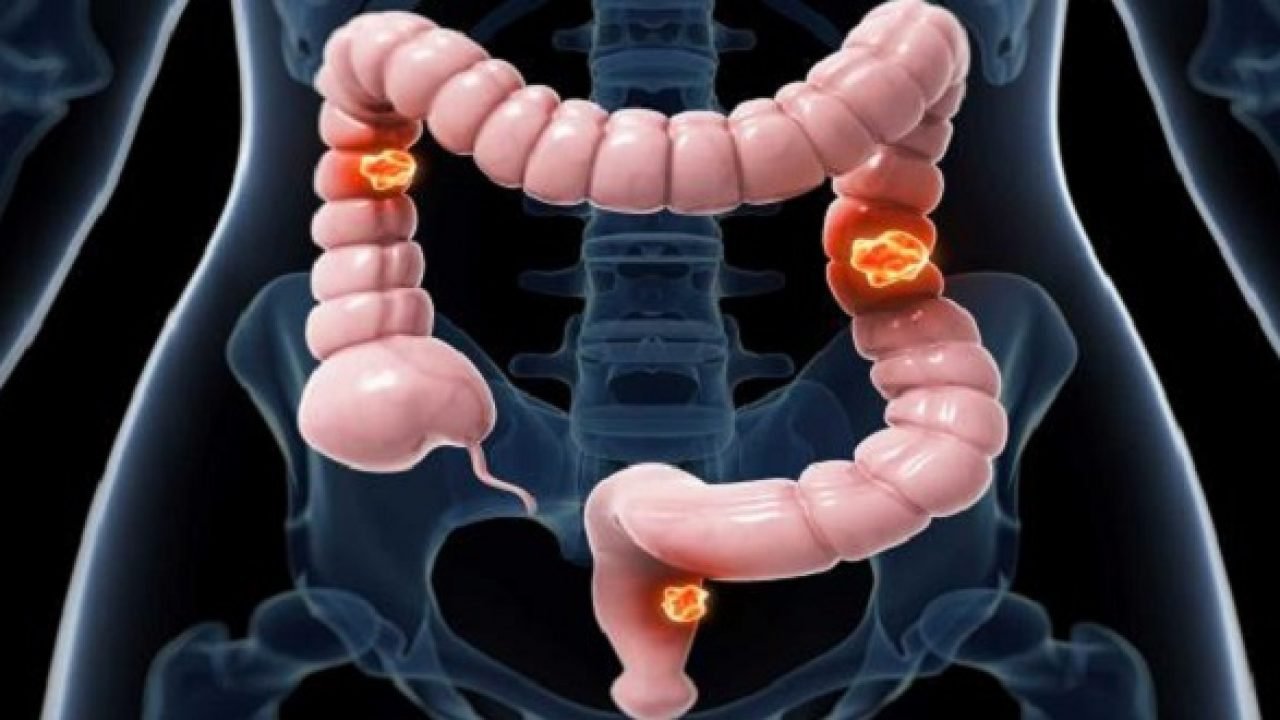
Thuật toán học sâu phân biệt những bệnh nhân có đáp ứng hoàn toàn với hóa trị liệu bổ trợ cho ung thư trực tràng tiến triển tại chỗ với độ chính xác 80%
The deep learning algorithm discriminated among patients who had a complete response to adjuvant chemotherapy for locally advanced rectal cancer with 80% accuracy. This technological aid may allow the selection of patients particularly in favor of conservative treatment rather than complete surgical resection. This is the first study to use a Deep Learning Algorithm to predict the overall pathological response after adjuvant chemotherapy in locally advanced rectal cancer.
9. Conclusion Artificial intelligence AI could represent an essential diagnostic method for endoscopists and gastroenterologists to treat patients accordingly and predict their clinical outcomes. Artificial intelligence appears to be particularly valuable in gastrointestinal endoscopy, to improve the detection of premalignant and malignant lesions, or inflammatory lesions, GI bleeding, and pancreatic pathologies. However, the current limitations of Artificial Intelligence include the lack of high-quality data sets for developing ML machine learning algorithms. Furthermore, an important piece of evidence used to build machine learning algorithms has only come from preclinical studies. As the science of AI is in development, current limitations must be considered as future challenges, so they are truly inherited in medical applications, including the ability to predict difficult to situations characterized by uncertainty. All in all, AI is revolutionizing technology and impacting other ethical aspects like replacing human work with machines, but this has always been an open question since the industrial revolution. What can be done is to foster mutual cooperation through gastrointestinal endoscopy applications, to mutually benefit from achievements in both scientific fields.
To proactively protect your health, you should have regular check-ups and colorectal cancer screening as soon as you have doubts. Vinmec provides you with comprehensive health care services such as: Colorectal cancer screening, General health check-up. You will have a comprehensive examination with leading specialists, experienced and good at expertise, especially imaging technicians who are professionally trained at home and abroad, helping to diagnose prediction with high accuracy. Equipment to support the examination are imported completely new and modern from the US, Singapore, Japan, Korea, Canada.
If you have a need to examine and use service packages at Vinmec, please register to book an appointment at the website or contact Vinmec's hotline for detailed advice.
9. Conclusion Artificial intelligence AI could represent an essential diagnostic method for endoscopists and gastroenterologists to treat patients accordingly and predict their clinical outcomes. Artificial intelligence appears to be particularly valuable in gastrointestinal endoscopy, to improve the detection of premalignant and malignant lesions, or inflammatory lesions, GI bleeding, and pancreatic pathologies. However, the current limitations of Artificial Intelligence include the lack of high-quality data sets for developing ML machine learning algorithms. Furthermore, an important piece of evidence used to build machine learning algorithms has only come from preclinical studies. As the science of AI is in development, current limitations must be considered as future challenges, so they are truly inherited in medical applications, including the ability to predict difficult to situations characterized by uncertainty. All in all, AI is revolutionizing technology and impacting other ethical aspects like replacing human work with machines, but this has always been an open question since the industrial revolution. What can be done is to foster mutual cooperation through gastrointestinal endoscopy applications, to mutually benefit from achievements in both scientific fields.
To proactively protect your health, you should have regular check-ups and colorectal cancer screening as soon as you have doubts. Vinmec provides you with comprehensive health care services such as: Colorectal cancer screening, General health check-up. You will have a comprehensive examination with leading specialists, experienced and good at expertise, especially imaging technicians who are professionally trained at home and abroad, helping to diagnose prediction with high accuracy. Equipment to support the examination are imported completely new and modern from the US, Singapore, Japan, Korea, Canada.
If you have a need to examine and use service packages at Vinmec, please register to book an appointment at the website or contact Vinmec's hotline for detailed advice.
Để đặt lịch khám tại viện, Quý khách vui lòng bấm số HOTLINE hoặc đặt lịch trực tiếp TẠI ĐÂY. Tải và đặt lịch khám tự động trên ứng dụng MyVinmec để quản lý, theo dõi lịch và đặt hẹn mọi lúc mọi nơi ngay trên ứng dụng.
References
Russell S , Norvig P. Artificial Intelligence: A Modern Approach, Global Edition. 3rd editon. London: Pearson, 2016. Colom R , Karama S, Jung RE, Haier RJ. Human intelligence and brain networks. Dialogs Clin Neurosci . 2010; 12 :489-501. [PubMed] Goodfellow I, Bengio Y, Courville A. Deep Learning. Cambridge: The MIT Press, 2016.
Russell S , Norvig P. Artificial Intelligence: A Modern Approach, Global Edition. 3rd editon. London: Pearson, 2016. Colom R , Karama S, Jung RE, Haier RJ. Human intelligence and brain networks. Dialogs Clin Neurosci . 2010; 12 :489-501. [PubMed] Goodfellow I, Bengio Y, Courville A. Deep Learning. Cambridge: The MIT Press, 2016.
Bài viết này được viết cho người đọc tại Sài Gòn, Hà Nội, Hồ Chí Minh, Phú Quốc, Nha Trang, Hạ Long, Hải Phòng, Đà Nẵng.