New applications of artificial intelligence in the field of digestion
The article is written by MSc, BS. Mai Vien Phuong, Department of Medical Examination & Internal Medicine - Vinmec Central Park International General Hospital
Artificial intelligence has the potential to be applied during colonoscopy to automatically detect colorectal polyps and distinguish between cancerous and noncancerous polyps, with the potential to improve detection rates. adenomas, the incidence varies widely among endoscopists when performing a colonoscopy examination.
1. Overview of artificial intelligence AI
Artificial intelligence (AI) enables machines to provide disruptive value in a number of industries and applications. Applications of AI techniques, especially machine learning and more recently deep learning, are growing in gastroenterology. Computer-aided diagnosis of upper gastrointestinal endoscopy is increasingly attracting attention for the automatic and accurate identification of dysplasia in Barrett's esophagus, as well as for the early detection of gastric cancers (GCs). , thus preventing malignancies in the esophagus and stomach. In addition, sophisticated neural network technology can accurately assess Helicobacter pylori (H. pylori) infection during standard endoscopy without biopsy, thereby reducing the risk of cancer stomach letter. Artificial intelligence has the potential to be applied during colonoscopy to automatically detect colorectal polyps and distinguish between cancerous and noncancerous polyps, with the potential to improve detection rates. adenomas, the incidence varies widely among endoscopists when performing a colonoscopy examination. In addition, AI allows to establish the feasibility of endoscopic polypectomy of large colon polyp lesions based on surface features and microvascular changes
2. Introduction to machine learning algorithms and deep learning
Machine learning (ML) and deep learning (DL) can be considered as subfields of artificial intelligence. ML is a form of artificial intelligence that can aid in decision-making, allowing improvements to applied algorithms without programming, including examining data and implementing descriptive models and models. predicted (Figure 1).
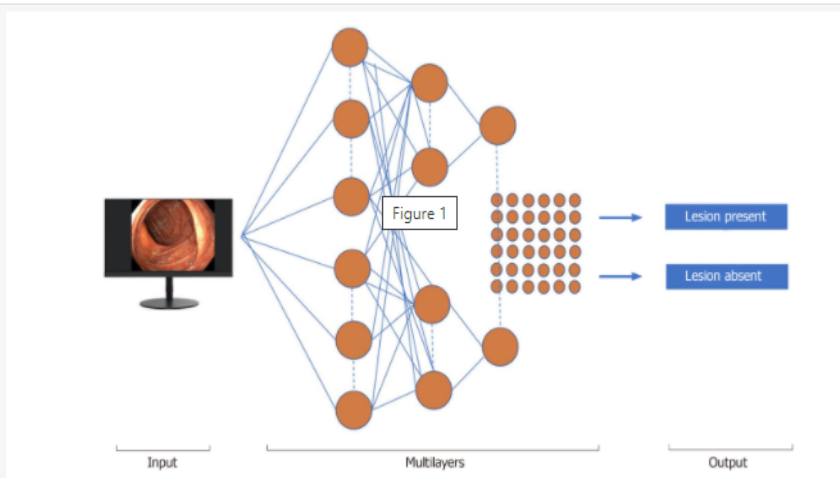
Mô hình ứng dụng trí tuệ nhân tạo trong nội soi tiêu hoá
Machine learning (ML) algorithms are differentiated into supervised and unsupervised methods. An example of supervised machine learning technology, an artificial neural network (ANN), mirrors the brain's schematic function. Each neuron is a computational unit and all neurons are connected to create a network. Machine learning algorithms and complex neural networks (CNNs) were created to train the software to distinguish between normal and abnormal regions in the intestinal lumen. To detect polyps, machine learning uses a fixed number of characteristics, such as the size, shape, and mucosal patterns of polyps.
3. Artificial intelligence, Barrett's esophagus and esophageal cancer
Barrett's esophagus (BE) is characterized by abnormal transformation (hyperplasia) of the cells of the mucosa, lining the lower part of the esophagus, from normally stratified squamous epithelium to columnar and associated epithelium. alternating cup-shaped cells. This condition represents a risk factor for esophageal adenocarcinoma (EAC) whose most severe prognosis is related to late diagnosis. Furthermore, 93% of patients can achieve complete remission after regular treatment and follow-up for 10 years. Promising techniques for the management of Barrett's esophagus, with the potential to reduce cancer risk by accurately diagnosing dysplasia, are under development.
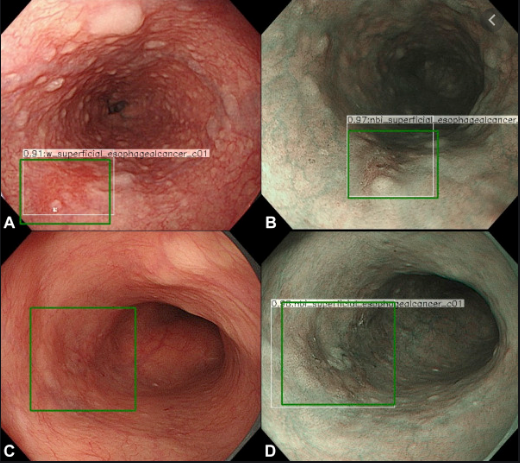
Sử dụng AI trong hệ thống nội soi: Ô vuông màu xanh là máy tính tự động khu trú hình ảnh tổn thương khi đưa máy soi đến.
Still based on the complex neural network system CNN and ResNet architecture with DeepLab V.3+, a modern reconfigured encoder-decoder network. To transmit the endoscope Livestream to our AI system, a capture card (Avermedia, Taiwan) for image transmission was integrated into the endoscope screen and the AI system was trained using 129 images. Endoscopic. All AI imaging findings were confirmed by pathological examination of resected samples of esophageal cancer as well as clamp biopsies (ie, Barrett's esophagus alone). The AI system showed a high performance score in lesion classification with sensitivity and specificity of 83.7% and 100%, respectively.
4. The role of the complex neural network CNN in the diagnosis of digestive pathology
The CNN complex neural network was also used by Horie et al., retrospectively collecting 8428 training images from esophageal cancer of 384 patients through the CNN complex neural network. CNN took 27 seconds to analyze 1118 test images and accurately detected cases of esophageal cancer with 98% sensitivity. The complex neural network system detects every 7 small cancerous lesions less than 10 mm in size. This system facilitates early and rapid malignancy detection leading to a better prognosis for these patients.
AI can assist endoscopists to do targeted biopsies with high accuracy, save work/time-consuming random sampling, with low sensitivity (64%) for detecting metaplasia. An international, randomized, crossover trial comparing high-definition white light endoscopy (HD-WLE) and NBI for the detection of IM and malignancy in 123 patients with BE (mean circumference and maximum size, 1.8 and 3.6 cm, respectively).
AI can assist endoscopists to do targeted biopsies with high accuracy, save work/time-consuming random sampling, with low sensitivity (64%) for detecting metaplasia. An international, randomized, crossover trial comparing high-definition white light endoscopy (HD-WLE) and NBI for the detection of IM and malignancy in 123 patients with BE (mean circumference and maximum size, 1.8 and 3.6 cm, respectively).
5. High Resolution Microscopic (at the cellular level) endoscopy (HRME)
High-resolution microscopic (at the cellular level) endoscopy (HRME) has shown the potential to enhance the detection of esophageal SCC during screening. An automated, real-time analysis algorithm was developed and evaluated using training and validation tests obtained from a previous in-vivo study involving 177 subjects. for screening/surveillance programs. In a post-analysis, the algorithm recognized malignancies with 95% sensitivity and 91% specificity, in the validation dataset, compared with 84% and 95% in the original study. The technology could therefore be applied in facilities where operators have less expertise in interpreting HRME images.
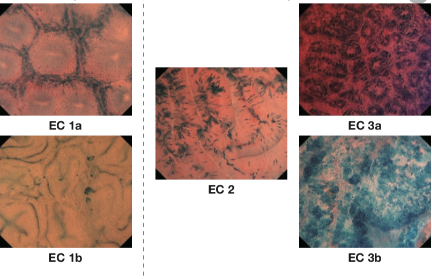
Hình ảnh nội soi phóng đại ở mức độ tế bào (nội soi tế bào)
6. Artificial Intelligence and Stomach Cancer
Gastric cancer (GC) is the third leading cause of death from malignancy worldwide, and gastroduodenal endoscopy (EGD) is considered the best diagnostic tool for cancer. at the early stage. The treatment of gastric tumor depends on the depth of the submucosal invasion; indeed, for differentiated intramucosal tumors (M) or those invading the superficial submucosa (≤ 500 lm: SM1), EMR mucosal resection or submucosal dissection ESD is performed to resect the lesion, while tumors with deep submucosal invasion (>500 lm:SM2) should be treated surgically to avoid the potential risk of local invasion and metastasis. Magnified endoscopy in combination with NBI or FICE (flexible color enhancement of spectral imaging) is clinically useful in distinguishing gastric malignancy from non-malignant regions. However, this optical diagnosis is completely dependent on the expertise and experience of the operator, which precludes its general use in clinical practice.
Miyaki et al., have developed software that allows quantitative assessment of mucosal GC on magnified gastrointestinal endoscopy images obtained with FICE. They adopted a feature framework featuring densely sampled scale-invariant feature descriptors to magnify the FICE images of 46 cases of intramucosal gastric cancer and then compared with histological findings. The CAD system enables detection accuracy of 86%, sensitivity and specificity of 85% and 87% for cancer diagnosis.
Miyaki et al., have developed software that allows quantitative assessment of mucosal GC on magnified gastrointestinal endoscopy images obtained with FICE. They adopted a feature framework featuring densely sampled scale-invariant feature descriptors to magnify the FICE images of 46 cases of intramucosal gastric cancer and then compared with histological findings. The CAD system enables detection accuracy of 86%, sensitivity and specificity of 85% and 87% for cancer diagnosis.
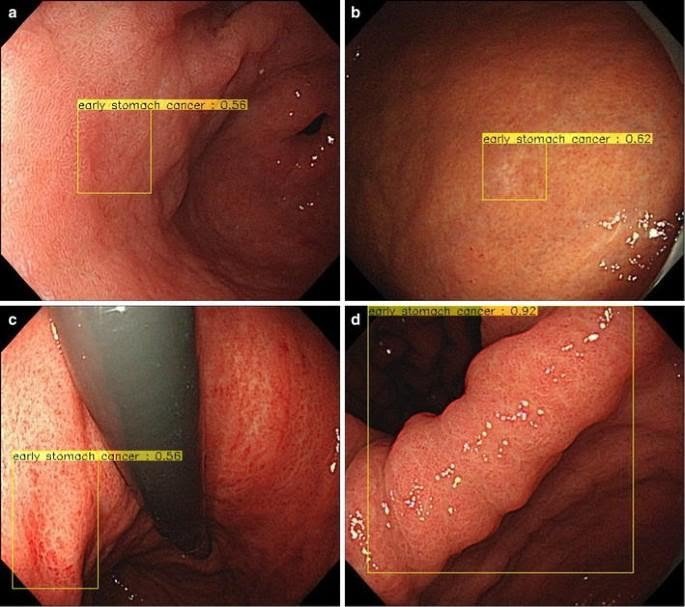
Tổn thương ung thư sớm dạ dày được phát hiện bằng trí tuệ nhân tạo
7. The role of AI in predicting the prognosis of patients with stomach cancer
AI can help doctors predict the prognosis of patients with stomach cancer. Several important clinical trials evaluating adjuvant strategies for highly advanced gastric cancer have been produced in the past decade, but the most appropriate therapy for gastric cancer to date remains uncertain. . In addition, two contemporary molecular landscape studies have demonstrated the presence of different molecular gastric cancer subtypes. A deep learning algorithm-based model (survival recurrence network, SRN) was developed to predict survival events for a total of 1190 gastric cancer patients, based on clinical/pathological data as well as as treatment protocol, predict outcomes at each time point during a 5-year monitoring period. The SRN indicates that the mesenchymal subtype of gastric cancer should stimulate an appropriate postoperative treatment strategy as a consequence of the enormous recurrence rate.
8. AI in identifying Helicobacter pylori infection
Helicobacter pylori (H. pylori) infects gastric epithelial cells and is associated with functional gastrointestinal disorders, peptic ulcers, mucosal atrophy, intestinal metaplasia, and gastric cancer. Chronic gastritis associated with H. pylori may also increase the risk of stomach cancer. CNN technology can accurately assess H. pylori infection during routine endoscopy without the need for biopsies. In an experimental study by Zheng et al., the authors introduced a Computer Aided Decision Support System that used CNN to estimate H. pylori infection based on endoscopic imaging. From 1959 patients, 77% were assigned to the confirmation group (1507 patients; 11729 gastric images) and 56% of them were infected with H. pylori (847), while 23% were selected to the confirmation group. (452) and 69% of patients with H. pylori infection (310; 3755 total images).
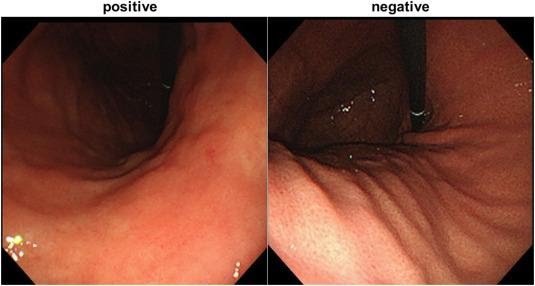
Hình ảnh nội soi đại diện của Helicobacter pylori dương tính và dạ dày âm tính. Teo và đỏ lan tỏa được nhìn thấy khi có nhiễm H.pylori. Một sự sắp xếp đều đặn của các tiểu tĩnh mạch thu thập (RAC) được nhìn thấy trong dạ dày không bị nhiễm H.pylori
9. Artificial intelligence AI for the diagnosis of polyps and colon cancer
Colorectal cancer (CRC) is the third most common malignancy in men and the second in women, and the fourth most common cause of cancer death. The National Polyp Study Registered that 70%-90% CRC can be prevented with routine endoscopic monitoring and polyp removal, but 7%-9% CRC can occur regardless of measures. this.
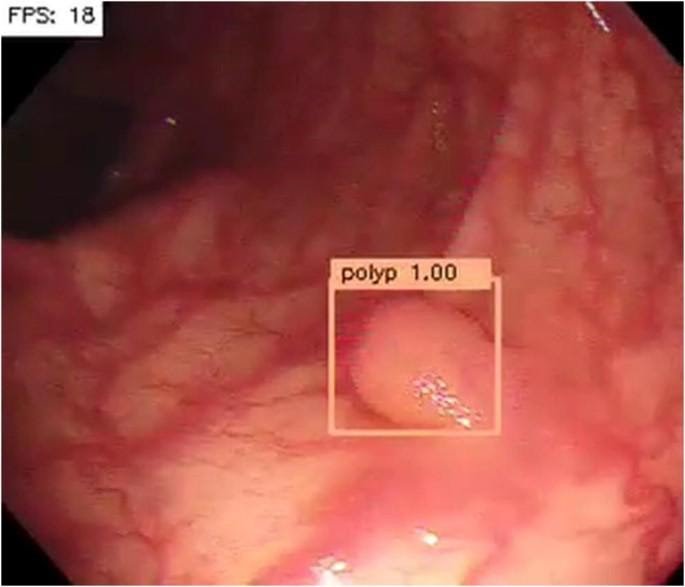
Hình ảnh nội soi có sử dụng trí tuệ nhân tạo: máy tính tự động tìm và định vị polyp, đánh giáp polyp đó bao nhiêu phần trăm ung thư
About 85% of "gap cancers" are caused by missed polyps or incomplete resection of polyps. Adenomas are the most common precancerous lesions throughout the colon. The ADR measures the endoscopist's ability to identify adenomas. ADRs range from 7%–53% among performing endoscopic surgeons depending on their qualifications, laparoscopic resection technique, time of withdrawal, quality of bowel preparation, and dependent determinants. into another process.
10. Several endoscopic improvements have been promoted to increase ADR
A review of 5 studies on the effects of high-resolution colonoscopy on ADR showed conflicting results; One study concluded that ADR was elevated exclusively for endoscopists with ADRs lower than 20%.
A prospective study of 55 patients used the prototype of a new automated polyp detection software (APDS) for automated image-based polyp detection and with overall polyp detection rates over time. actually 75%. Smaller polyp size and flat polyp morphology are associated with APDS not detecting enough polyps.
A prospective study of 55 patients used the prototype of a new automated polyp detection software (APDS) for automated image-based polyp detection and with overall polyp detection rates over time. actually 75%. Smaller polyp size and flat polyp morphology are associated with APDS not detecting enough polyps.
11. Artificial intelligence helps distinguish between adenomas and hyperplastic polyps
In addition to the CADe machine, the CADx system is also used to differentiate between adenomas and hyperplastic polyps.
Byrne et al suggested the use of computerized image analysis to reduce variability in endoscopic findings and histological predictions. This AI model was trained using endoscopic video and was able to distinguish between small adenomas and hyperplastic polyps with high accuracy. In addition, it predicted histology with 94% accuracy, 98% sensitivity, 83% specificity, and negative and positive predictive values of 97% and 90%, respectively.
Hotta et al aimed to confirm the effectiveness of the endoscopic (EC)-CAD system in the diagnosis of malignant or non-malignant colorectal lesions, by comparing the diagnostic capabilities among specialist endoscopists. professionals and non-experts, using web-based tests. A confirmatory test was performed using endoscopic images of 100 small (<10 mm) colorectal lesions. The diagnostic accuracy and sensitivity of EB-01 and non-specialists for stained endoscopic images was 98.0% versus 69.0%, indicating significantly higher diagnostic accuracy and sensitivity for with non-specialist endoscopists when diagnosing small colorectal lesions.
The deep learning algorithm also helps distinguish patients who have a complete response to adjuvant chemotherapy for locally advanced rectal cancer with 80% accuracy. This technological aid may allow the selection of patients particularly in favor of conservative treatment rather than complete surgical resection. This is the first study to use a Deep Learning Algorithm to predict the overall pathological response after adjuvant chemotherapy in locally advanced rectal cancer.
Byrne et al suggested the use of computerized image analysis to reduce variability in endoscopic findings and histological predictions. This AI model was trained using endoscopic video and was able to distinguish between small adenomas and hyperplastic polyps with high accuracy. In addition, it predicted histology with 94% accuracy, 98% sensitivity, 83% specificity, and negative and positive predictive values of 97% and 90%, respectively.
Hotta et al aimed to confirm the effectiveness of the endoscopic (EC)-CAD system in the diagnosis of malignant or non-malignant colorectal lesions, by comparing the diagnostic capabilities among specialist endoscopists. professionals and non-experts, using web-based tests. A confirmatory test was performed using endoscopic images of 100 small (<10 mm) colorectal lesions. The diagnostic accuracy and sensitivity of EB-01 and non-specialists for stained endoscopic images was 98.0% versus 69.0%, indicating significantly higher diagnostic accuracy and sensitivity for with non-specialist endoscopists when diagnosing small colorectal lesions.
The deep learning algorithm also helps distinguish patients who have a complete response to adjuvant chemotherapy for locally advanced rectal cancer with 80% accuracy. This technological aid may allow the selection of patients particularly in favor of conservative treatment rather than complete surgical resection. This is the first study to use a Deep Learning Algorithm to predict the overall pathological response after adjuvant chemotherapy in locally advanced rectal cancer.
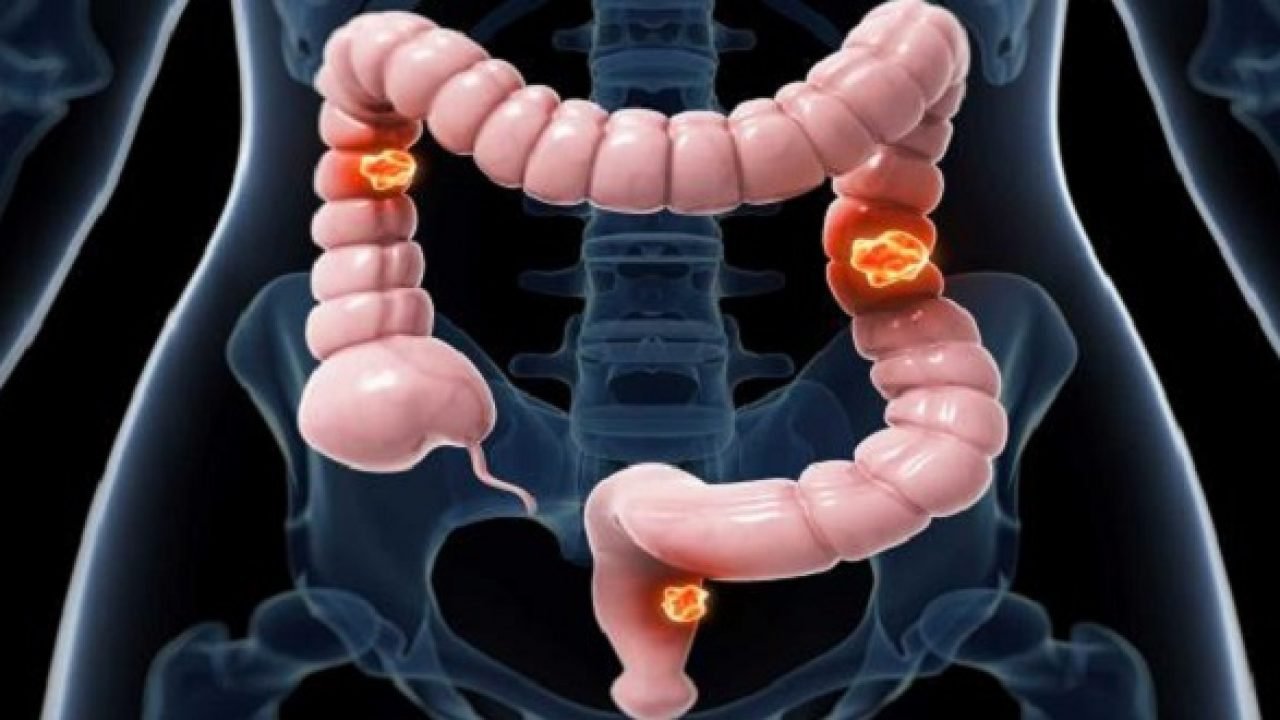
Thuật toán học sâu còn giúp phân biệt những bệnh nhân có đáp ứng hoàn toàn với hóa trị liệu bổ trợ cho ung thư trực tràng tiến triển tại chỗ với độ chính xác 80%
12. Some limits of artificial intelligence
Artificial intelligence AI could represent an essential diagnostic method for endoscopists and gastroenterologists to treat patients accordingly and predict their clinical outcomes. Artificial intelligence appears to be particularly valuable in gastrointestinal endoscopy, to improve the detection of premalignant and malignant lesions, or inflammatory lesions, GI bleeding, and pancreatic pathologies. However, the current limitations of Artificial Intelligence include the lack of high-quality data sets for developing ML machine learning algorithms. Furthermore, an important piece of evidence used to build machine learning algorithms has only come from preclinical studies. Potential selection biases cannot be ruled out in such cases. In this context, it is necessary to closely validate the performance of AI before using it in daily clinical practice. A true measure of AI accuracy, which should include as a side effect in overshoots and spectral biases. Overfitting occurs when a learning model self-adjusts too much on the training dataset and the predictions do not generalize well to the new data set. This effect clearly contradicts Occam's razor's problem-solving principle, which states that simpler theories have higher predictive quality. In the worst case of AI algorithm application, underfit can occur, obtaining models that cannot accurately demonstrate the underlying structure of the data set, thus also obtaining features of the predictive model is not good.
On the other hand, spectral bias occurs when the data set used to develop the model is not representative of the target population. To avoid overestimating accuracy and generality, it is important to ensure that an external validation dataset is collected in a way that minimizes spectral bias. In addition, well-designed multicenter observational studies are needed for more definitive confirmation. Certainly, there is also a need to acknowledge the ethical issues as the AI is not aware of the patient's choices or liabilities. Privacy issues can be solved using linked datasets that do not involve centralized servers. Future randomized studies may directly increase the overall value (quality versus cost) of CNN by examining its impact on surveillance colonoscopy, colonoscopy duration, polyps and ADRs, and pathological costs. As the science of AI is in development, current limitations must be considered as future challenges, so they are truly inherited in medical applications, including the ability to predict difficult to situations characterized by uncertainty. All in all, AI is revolutionizing technology and impacting other ethical aspects like replacing human work with machines, but this has always been an open question since the industrial revolution. What can be done is to foster mutual cooperation through gastrointestinal endoscopy applications, to mutually benefit from achievements in both scientific fields.
To actively protect health, subjects over 40 years old with a history of atrophic gastritis, chronic gastritis; Barrett's esophagus; submucosal tumors need periodic examination; People with anemia of unknown cause, after gastrectomy, family history of stomach cancer should be screened for gastrointestinal cancer periodically. Currently, Vinmec offers a package of screening and early detection of cancers of the gastrointestinal tract (esophagus - stomach - colon) combining clinical and paraclinical examination to bring about the most accurate results possible. With this package, you will get:
Gastroenterology Specialist Examination; Gastroscopy and colonoscopy with NBI endoscope under anesthesia; Gastroscopy and colonoscopy with anesthesia; Routine histopathological examination of fixation, transfer, casting, cutting, staining... of biopsies (upper gastrointestinal tract (esophagus, stomach, duodenum, papilla) through endoscopic examination; Histopathological examination Routinely fix, transfer, cast, cut, dye... biopsies (lower gastrointestinal tract through endoscopy (colon, rectum). Vinmec, please register to book an appointment right at the website or contact Vinmec's hotline for detailed advice.
On the other hand, spectral bias occurs when the data set used to develop the model is not representative of the target population. To avoid overestimating accuracy and generality, it is important to ensure that an external validation dataset is collected in a way that minimizes spectral bias. In addition, well-designed multicenter observational studies are needed for more definitive confirmation. Certainly, there is also a need to acknowledge the ethical issues as the AI is not aware of the patient's choices or liabilities. Privacy issues can be solved using linked datasets that do not involve centralized servers. Future randomized studies may directly increase the overall value (quality versus cost) of CNN by examining its impact on surveillance colonoscopy, colonoscopy duration, polyps and ADRs, and pathological costs. As the science of AI is in development, current limitations must be considered as future challenges, so they are truly inherited in medical applications, including the ability to predict difficult to situations characterized by uncertainty. All in all, AI is revolutionizing technology and impacting other ethical aspects like replacing human work with machines, but this has always been an open question since the industrial revolution. What can be done is to foster mutual cooperation through gastrointestinal endoscopy applications, to mutually benefit from achievements in both scientific fields.
To actively protect health, subjects over 40 years old with a history of atrophic gastritis, chronic gastritis; Barrett's esophagus; submucosal tumors need periodic examination; People with anemia of unknown cause, after gastrectomy, family history of stomach cancer should be screened for gastrointestinal cancer periodically. Currently, Vinmec offers a package of screening and early detection of cancers of the gastrointestinal tract (esophagus - stomach - colon) combining clinical and paraclinical examination to bring about the most accurate results possible. With this package, you will get:
Gastroenterology Specialist Examination; Gastroscopy and colonoscopy with NBI endoscope under anesthesia; Gastroscopy and colonoscopy with anesthesia; Routine histopathological examination of fixation, transfer, casting, cutting, staining... of biopsies (upper gastrointestinal tract (esophagus, stomach, duodenum, papilla) through endoscopic examination; Histopathological examination Routinely fix, transfer, cast, cut, dye... biopsies (lower gastrointestinal tract through endoscopy (colon, rectum). Vinmec, please register to book an appointment right at the website or contact Vinmec's hotline for detailed advice.
Để đặt lịch khám tại viện, Quý khách vui lòng bấm số HOTLINE hoặc đặt lịch trực tiếp TẠI ĐÂY. Tải và đặt lịch khám tự động trên ứng dụng MyVinmec để quản lý, theo dõi lịch và đặt hẹn mọi lúc mọi nơi ngay trên ứng dụng.
References
Russell S , Norvig P. Artificial Intelligence: A Modern Approach, Global Edition. 3rd editon. London: Pearson, 2016. Colom R , Karama S, Jung RE, Haier RJ. Human intelligence and brain networks. Dialogs Clin Neurosci . 2010; 12 :489-501. [PubMed] Goodfellow I, Bengio Y, Courville A. Deep Learning. Cambridge: The MIT Press, 2016. Pohl H , Welch HG. The role of overdiagnosis and reclassification in the marked increase of esophageal adenocarcinoma incidence. J Natl Cancer Inst . 2005; 97 :142-146. [PubMed] [DOI] Dent J . Barrett's esophagus: A historical perspective, an update on core practicalities and predictions on future evolutions of management. J Gastroenterol Hepatol . 2011; 26 Suppl 1:11-30. [PubMed] [DOI] Gaetano Cristian Morreale, Emanuele Sinagra, et al., Emerging artificial intelligence applications in gastroenterology: A review of the literature, Artif Intell Gastrointest Endosc. Jul 28, 2020; 1(1): 6-18
Russell S , Norvig P. Artificial Intelligence: A Modern Approach, Global Edition. 3rd editon. London: Pearson, 2016. Colom R , Karama S, Jung RE, Haier RJ. Human intelligence and brain networks. Dialogs Clin Neurosci . 2010; 12 :489-501. [PubMed] Goodfellow I, Bengio Y, Courville A. Deep Learning. Cambridge: The MIT Press, 2016. Pohl H , Welch HG. The role of overdiagnosis and reclassification in the marked increase of esophageal adenocarcinoma incidence. J Natl Cancer Inst . 2005; 97 :142-146. [PubMed] [DOI] Dent J . Barrett's esophagus: A historical perspective, an update on core practicalities and predictions on future evolutions of management. J Gastroenterol Hepatol . 2011; 26 Suppl 1:11-30. [PubMed] [DOI] Gaetano Cristian Morreale, Emanuele Sinagra, et al., Emerging artificial intelligence applications in gastroenterology: A review of the literature, Artif Intell Gastrointest Endosc. Jul 28, 2020; 1(1): 6-18